Understanding Annotation in Machine Learning: A Pillar of Software Development
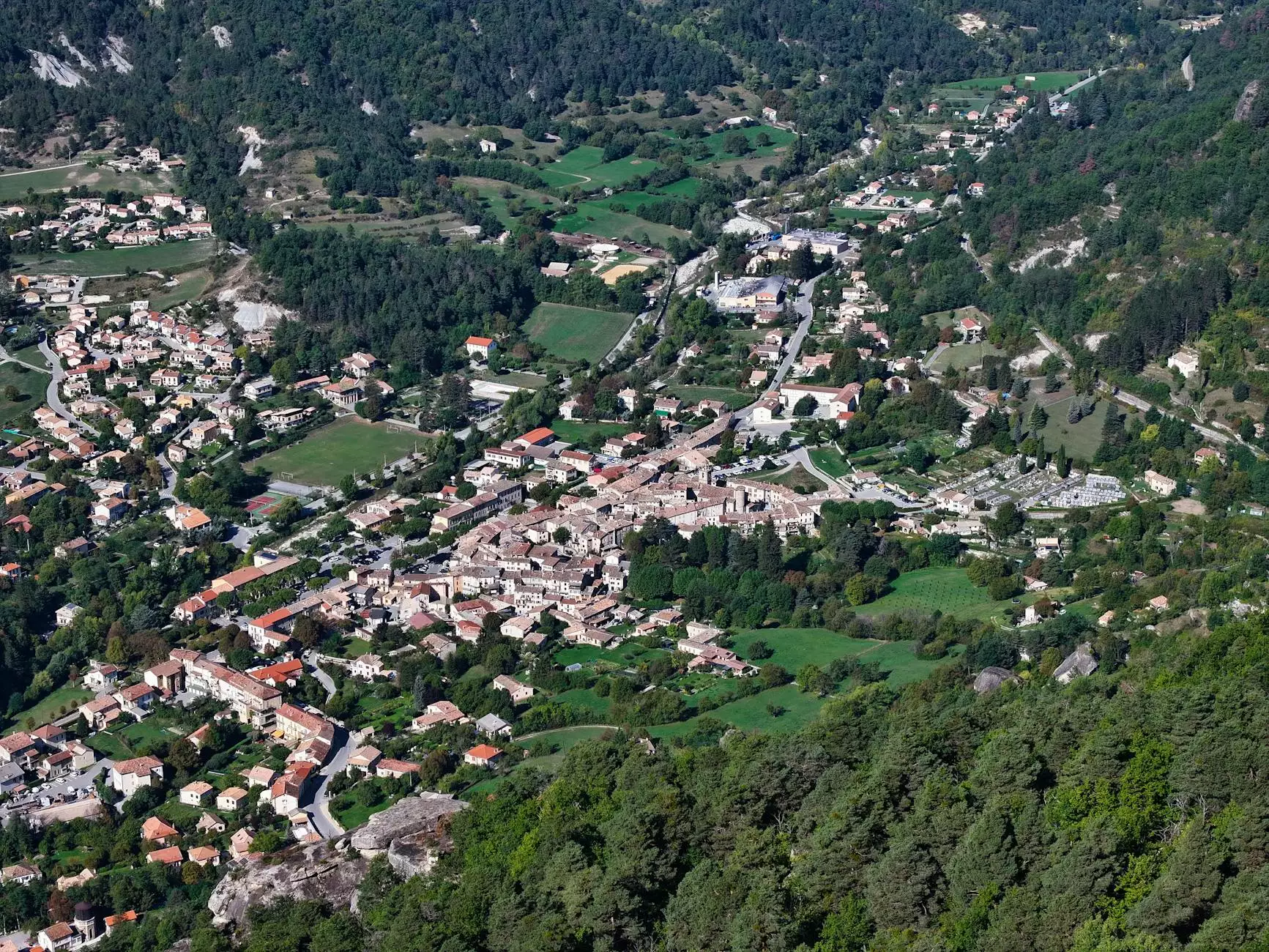
In the rapidly evolving world of technology, the importance of annotation machine learning cannot be overstated. This critical process serves as a backbone to developing effective and efficient machine learning models. At Keymakr, we recognize how integral this process is for businesses wishing to harness the power of artificial intelligence (AI) and big data analytics.
What is Annotation Machine Learning?
Annotation machine learning involves the act of labeling or tagging data to provide meaningful context that machine learning algorithms require for training. This annotated data helps algorithms understand input features, making it possible for them to draw accurate conclusions and predictions.
Examples include:
- Image Recognition: Annotating images with labels pertaining to their content, like 'dog', 'cat', or 'car'.
- Text Classification: Labeling texts based on sentiment like 'positive', 'negative', or 'neutral'.
- Object Detection: Identifying objects in images with bounding boxes and corresponding labels.
The Importance of Annotation in Machine Learning
In machine learning, quality data is paramount. Unannotated or poorly annotated data can lead to inaccurate models which compromises the value derived from these systems. Here are several critical reasons why annotation is vital:
- Enhanced Accuracy: Accurate annotations lead to greater precision in model predictions.
- Improved Model Training: Annotated datasets provide a robust framework for training algorithms.
- Domain-Specific Applications: Different industries require specialized annotations, enhancing the model's effectiveness in those areas.
Types of Annotation Methods
There are various methods for annotating data, each tailored to specific machine learning requirements:
- Video Annotation: Involves creating labels for sequences within video datasets.
- Audio Annotation: Used for speech recognition tasks, including transcribing and labeling audio clips.
- Text Annotation: Involves tagging parts of speech and sentiments in texts, crucial for natural language processing (NLP).
How Keymakr Excels in Annotation Machine Learning
At Keymakr, we adopt a systematic approach to annotation machine learning. Our methodologies ensure high-quality data annotation that fuels the development of robust AI models. Here’s how we make a difference:
1. Utilizing Advanced Annotation Tools
Our platform integrates cutting-edge technologies designed to streamline data annotation. Keymakr’s tools facilitate efficient annotation workflows, reducing time while enhancing output accuracy.
2. Skilled Annotation Teams
Our team consists of trained professionals who understand the nuances of annotation across various domains, ensuring the annotations are contextually relevant and precise.
3. Customizable Annotation Solutions
We recognize that every business is unique. Our services offer customizable solutions to align with specific project requirements, enabling us to cater to diverse industries.
Application of Annotation Machine Learning Across Industries
Annotation machine learning finds applications in numerous sectors, each leveraging it to enhance operations:
1. Healthcare
In healthcare, annotated data is used to assist in diagnostics through image recognition, enabling systems to detect anomalies in medical scans.
2. Automotive
The automotive industry utilizes annotation machine learning for developing autonomous vehicles. Annotating images and videos helps algorithms understand road scenarios, objects, and obstacles.
3. Retail
Retailers employ annotated data for customer behavior analysis, which enhances personalized marketing strategies through effective segmentation.
Challenges in Annotation Machine Learning
Despite its benefits, the annotation process poses several challenges that businesses must navigate:
- High Costs: Annotating large datasets can be resource-intensive, requiring financial investment in tools and skilled personnel.
- Time-Consuming: Quality annotation is a meticulous process that often takes considerable time, which can hinder project timelines.
- Consistency Issues: Ensuring consistency across annotations performed by multiple annotators can be difficult, potentially affecting model performance.
The Future of Annotation Machine Learning
The future of annotation in machine learning looks promising. As AI technologies advance, we can expect innovations that will streamline the annotation process. Potential trends include:
- Automation: Increased automation through AI will reduce manual input in the annotation process, leading to enhanced efficiencies.
- Crowdsourcing: Leveraging crowdsourced platforms for mass data annotation can expedite processes while potentially lowering costs.
- Integration of Active Learning: Active learning approaches allow algorithms to identify which data points require annotation, improving efficiency.
Conclusion
In conclusion, annotation machine learning is vital for any organization looking to implement effective AI solutions. At Keymakr, our commitment to superior data annotation ensures that businesses can harness the full potential of machine learning technologies. With applications spanning across various industries and a future that promises more innovations, engaging with expert annotation services can chart a path toward success.
Whether you're in software development, healthcare, retail, or any other sector, investing in quality annotation will empower your business's AI initiatives. Embrace the change and join the wave of businesses leveraging advanced annotation machine learning solutions.