Labeling Tools for Machine Learning: A Comprehensive Guide
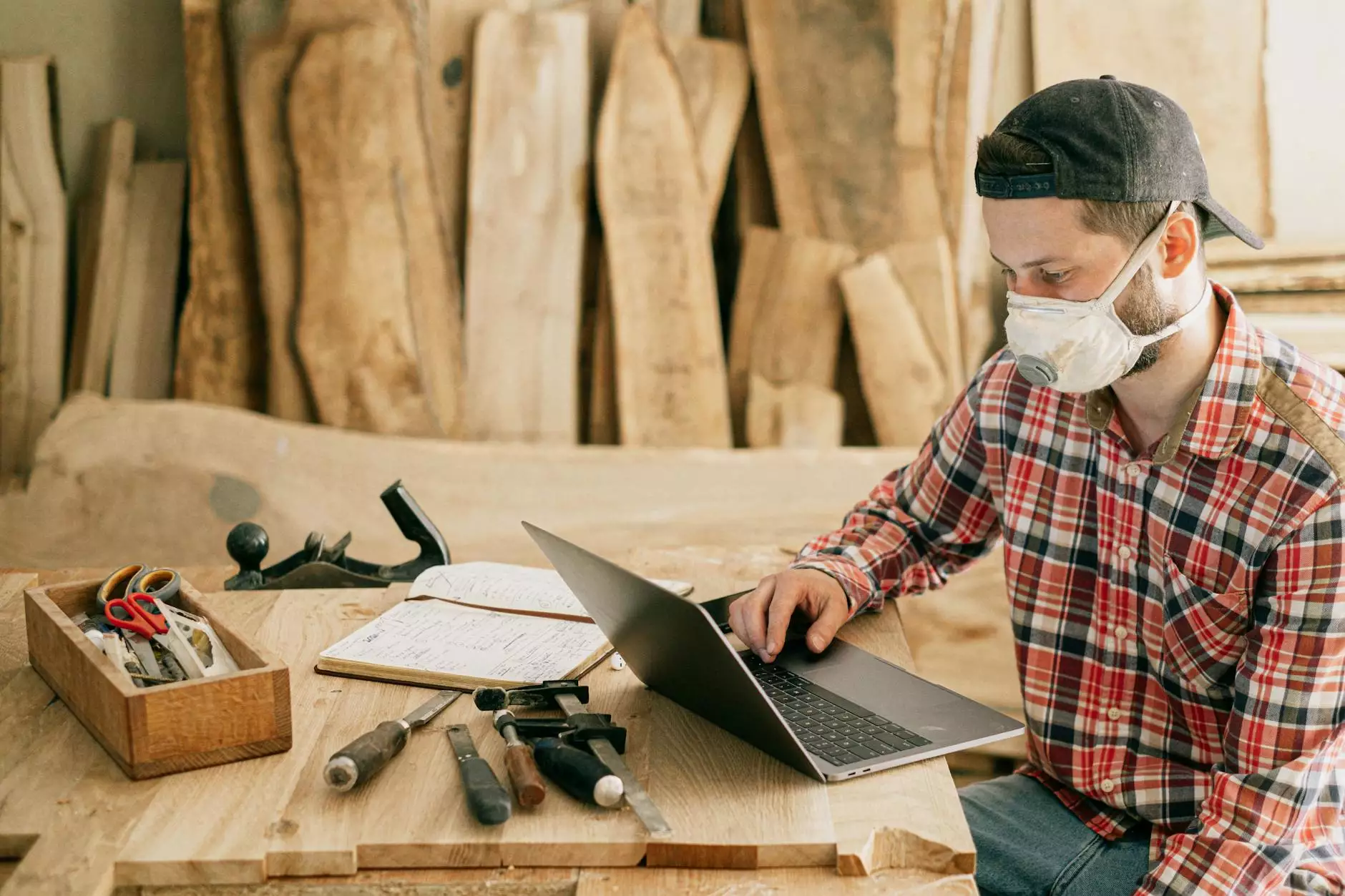
In the rapidly evolving world of machine learning, the importance of quality data cannot be overstated. One of the critical components of data quality is effective and accurate labeling tools for machine learning. This article will explore these tools, the significance of data annotation, and how organizations like KeyLabs.ai are leading the charge in providing top-notch data annotation tools.
Understanding Data Annotation
Data annotation is the process of labeling datasets to make them recognizable to machine learning algorithms. Without this fundamental process, machines would lack the capability to learn from the data presented, making data annotation essential for the success of any AI project.
The Role of Data Annotation in Machine Learning
Machine learning involves teaching algorithms to recognize patterns based on input data. This process requires:
- Labeling Data: Assigning meaningful tags to data points.
- Improving Model Accuracy: Enhancing algorithm performance through high-quality labeled data.
- Facilitating Supervised Learning: Training models with annotated datasets to predict outcomes.
Types of Data Annotation
There are various types of data annotation based on the data format:
- Image Annotation: Labeling objects within images for tasks like object detection.
- Text Annotation: Tagging sections of text for tasks like sentiment analysis.
- Video Annotation: Identifying actions or objects in video frames.
- Audio Annotation: Labeling audio clips for speech recognition tasks.
Each of these categories requires specialized labeling tools for machine learning to ensure accuracy and efficiency in the annotation process.
Why Use Labeling Tools for Machine Learning?
Choosing the right labeling tools for machine learning can significantly impact your project’s outcome. Here are several reasons why they are essential:
- Consistency: Automated tools ensure that the labeling remains consistent, reducing variations that can confuse the learning algorithm.
- Speed: Labeling tools can process large volumes of data much faster than manual methods.
- Better Quality: Advanced tools often incorporate review systems that enhance the quality of annotations.
- Scalability: As your data needs grow, so too can your labeling tool’s capabilities.
Choosing the Right Labeling Tool
When it comes to selecting the right labeling tools for machine learning, it’s essential to consider various factors:
1. Type of Data Supported
Ensure that the tool can handle the specific data formats you are working with, whether it's images, text, videos, or audio.
2. User Interface and Ease of Use
A user-friendly interface means that your team can spend less time learning the tool and more time annotating data.
3. Customization Options
The ability to customize labeling categories and workflows can greatly enhance your team’s productivity.
4. Quality Control Features
Look for tools that offer built-in quality checks and the ability to review annotations easily.
5. Integration Capabilities
Your labeling tool should be able to integrate with your existing data pipelines and machine learning frameworks seamlessly.
KeyLabs.ai: Leading the Charge in Data Annotation
KeyLabs.ai specializes in providing comprehensive data annotation tools that empower businesses to annotate data efficiently and effectively. Here’s how they stand out:
Advanced Technology
KeyLabs.ai utilizes cutting-edge technology to improve the accuracy and speed of the data annotation process. Their tools incorporate AI and machine learning capabilities to assist human annotators, ensuring high-quality outputs.
Expert Workforce
The team at KeyLabs.ai is composed of skilled professionals with expertise in various domains, providing an additional layer of quality assurance to ensure the labels assigned are contextually relevant and accurate.
Robust API and Integrations
KeyLabs.ai’s labeling tools come with robust APIs that allow organizations to integrate them into their workflows easily. This capability is crucial for businesses looking to scale their machine learning projects.
The Future of Labeling Tools for Machine Learning
As machine learning continues to evolve, so too will the labeling tools for machine learning. Here are some anticipated trends:
- Increased Automation: The rise of AI-powered labeling tools will continue to automate repetitive tasks and reduce human error.
- Greater Collaboration: Tools that allow for real-time collaboration among team members will become more popular as remote work expands.
- Enhanced Training Models: With more diverse data sources, labeling tools will be designed to support multi-modal annotations, enabling more complex machine learning applications.
In conclusion, the world of machine learning is heavily reliant on the quality of data, and at the heart of this data is effective labeling. Using cutting-edge labeling tools for machine learning, such as those offered by KeyLabs.ai, organizations can ensure that they have the quality data necessary to train robust machine learning models.
Investing in superior data annotation tools not only streamlines the annotation process but also paves the way for innovative advancements in technology. As businesses continue to embrace artificial intelligence, the demand for accurate, scalable, and efficient labeling solutions will only grow, making it imperative to stay ahead of the curve.
Conclusion
In a data-driven world, the significance of labeling tools for machine learning cannot be ignored. By utilizing top-tier platforms like KeyLabs.ai, organizations are not just annotating data; they are investing in the future of artificial intelligence. To succeed in this competitive landscape, ensure your data annotation processes are equipped with the best tools available, and reap the rewards of enhanced model performance, accuracy, and efficiency.